CSE 6250: Big Data for Health Informatics
Instructional Team
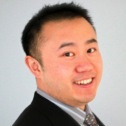
Jimeng Sun
Creator, Instructor
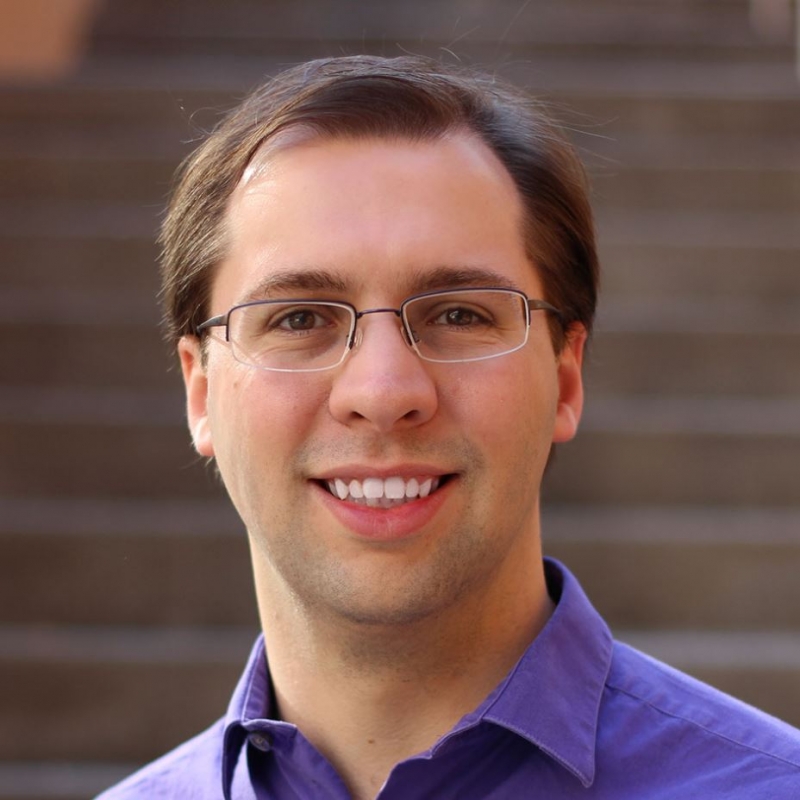
David Joyner
Course Developer
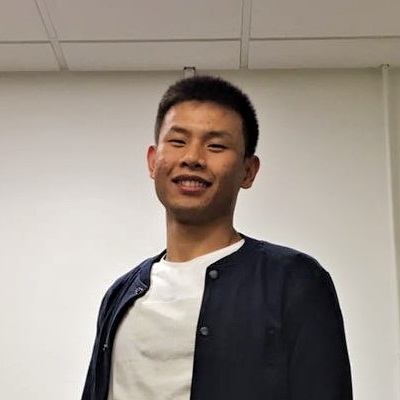
Quan Guo
Head TA
Overview
Data science plays an important role in many industries. In facing massive amounts of heterogeneous data, scalable machine learning and data mining algorithms and systems become extremely important for data scientists. The growth of volume, complexity, and speed in data drives the need for scalable data analytic algorithms and systems. In this course, we study such algorithms and systems in the context of healthcare applications.
In healthcare, large amounts of heterogeneous medical data have become available in various healthcare organizations (payers, providers, pharmaceuticals). Those data could be an enabling resource for deriving insights for improving care delivery and reducing waste. The enormity and complexity of these datasets present great challenges in analyses and subsequent applications to a practical clinical environment.
In this course, we introduce the characteristics of medical data and associated data mining challenges on dealing with such data. We cover various algorithms and systems for big data analytics. We focus on studying those big data techniques in the context of concrete healthcare analytic applications such as predictive modeling, computational phenotyping, and patient similarity.
We also study big data analytic technology:
- Scalable machine learning algorithms such as online learning and fast similarity search
- Big data analytic systems including Hadoop family (Hive, Pig, HBase), Spark, and Graph DB
More information is available on the CSE 6250 course website. Note that the textbook Introduction to Deep Learning for Healthcare by Cao Xiao and Jimeng Sun is required for this course.
![]() |
![]() |
Preview
Sample Syllabi
Spring 2024 syllabus (PDF)
Spring 2023 syllabus (PDF)
Fall 2022 syllabus (PDF)
Note: Sample syllabi are provided for informational purposes only. For the most up-to-date information, consult the official course documentation.
Course Content
To access the public version of this course's content, click here, then log into your Ed Lessons account. If you have not already created an Ed Lessons account, enter your name and email address, then click the activation link sent to your email, then revisit that link.
Before Taking This Class...
Suggested Background Knowledge
- CS 7641: Machine Learning course; of particular importance are machine learning and data mining concepts such as classification and clustering
- Proficient programming and system skills in Scala, Python, and Java
- Proficient knowledge and experience in dealing with data; understanding of the ETL process (recommended skills include SQl, NoSQL such as MongoDB)
- Minimum grade of C for MATH 3215 or MATH 3225 or ECE 3077 or ISYE 2027
- Two of the following:
- CX 4240: Introduction to Computing for Data Analysis
- CS 4400: Introduction to Database Systems
- CX 4242: Data and Visual Analytics
Technical Requirements and Software
- Browser and connection speed: An up-to-date version of Chrome, Firefox, or Internet Explorer is strongly recommended. 2+ Mbps is recommended; the minimum requirement is 1 Mbps download speed.
- Operating system:
- PC: Windows XP or higher with latest updates installed
- Mac: OS X 10.6 or higher with latest updates installed
- Linux: any recent distribution that has the supported browsers installed
- Cloud Computing: Amazon Web Service (AWS) or MS Azure
- Virtual Machine: Docker or other virtual machine will be needed
Academic Integrity
All Georgia Tech students are expected to uphold the Georgia Tech Academic Honor Code. This course may impose additional academic integrity stipulations; consult the official course documentation for more information.